The shift from traditional Private Equity to data-driven decisions
Private equity (PE) has traditionally operated in a discreet relationship-based model compared to public markets. While trust and industry expertise have been at the heart of deal sourcing and decision-making, recent advancements in AI and data science are transforming how private equity firms approach deal sourcing, due diligence, and market assessment.
PE firms are beginning to explore ways to incorporate AI-driven strategies and automate their processes. AI can offer three distinct opportunities for PE firms: automating back-office tasks (such as document verification, summarizing notes, etc.), enabling data-driven investing, and enhancing portfolio management through digitalization. This article focuses on how AI can help funds make better, data-driven decisions.
While public markets can leverage vast amounts of data and quantitative analysis in a more transparent environment, AI for private equity must retain a careful balance between automation and human insight due to its relationship-focused nature.
The role of AI in strategic decision-making: widening the deal funnel
Missing a valuable deal or market opportunity is one of the greatest fears for any PE firm. While PE deals have always been supported by large amounts of data, this data is traditionally utilized in a post-talk manner —meaning after discussions and initial assessments have already occurred. AI offers a way to make these processes more data-driven and efficient by automating repetitive tasks and providing more precise, real-time data insights. Besides, AI tools can mitigate this risk by widening the funnel of deal sourcing and making the decision-making process more systematic and data-driven.
At a strategic level, AI enables PE firms to leverage a broad range of structured and unstructured data to answer critical questions about market growth, sub-market trends, and investment potential. One area of focus is the implementation of predictive analytics to monitor market changes and anticipate emerging trends. By feeding real-time data into machine learning models, firms can create early-warning systems that detect sectoral changes and business vulnerabilities. This proactive approach allows firms to either capitalize on growing sectors or exit declining ones in a timely manner. The ability to integrate AI at this strategic level helps firms “see all the things they should see”—preventing missed opportunities and offering a clearer picture of which markets are likely to grow over time. It’s a shift toward a more calculated and comprehensive investment approach.
Real-world implementation for data-driven decisions
One of the most significant benefits of AI in deal sourcing is its ability to widen the opportunity funnel. By systematically structuring and analyzing vast amounts of public and private data, AI can identify potentially lucrative deals that might have gone unnoticed. This not only broadens the scope of deal sourcing but also helps in optimizing analysts’ time and resources.
For instance, a combination of data scientists, machine learning engineers, and industry experts can collaborate to build AI models that evaluate companies, sectors, and markets based on growth potential and risk factors. Over time, these models can gain accuracy and reliability, enhancing the firm’s confidence in making data-backed decisions.
Below is an example of a data-driven process for deal discovery and investing:
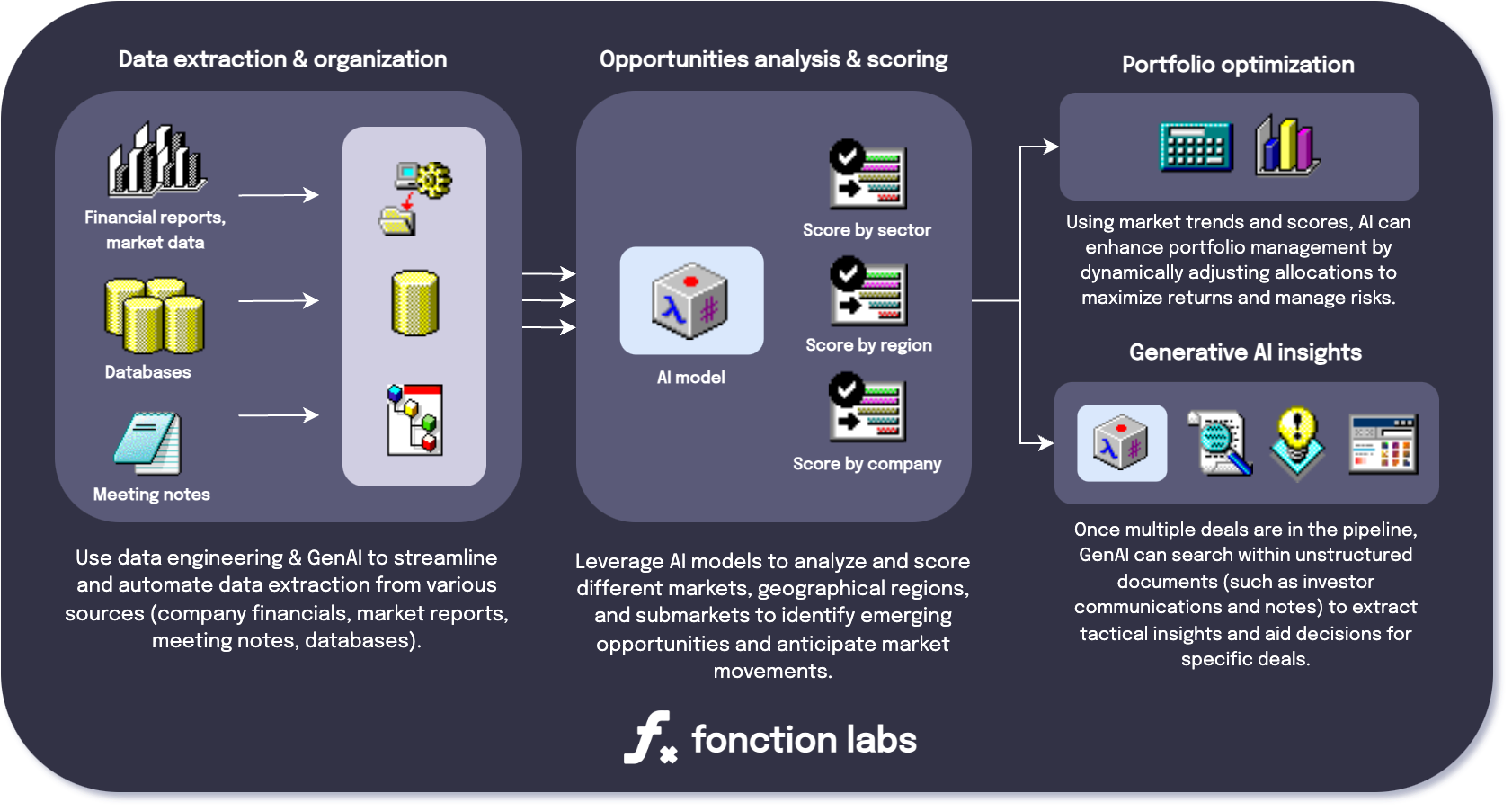
-
Data extraction & organization: Use data engineering and generative AI (GenAI) to automate data extraction from various sources such as company financials, market reports, meeting notes, and databases. This automation enables analysts to focus on strategic assessments while ensuring comprehensive coverage of critical information.
-
Opportunities analysis and scoring: Leverage AI algorithms to analyze and score different markets, geographical regions, and submarkets. These models can identify emerging opportunities, anticipate market movements, and highlight promising deals. This approach adds an additional layer of foresight, beyond human intuition or isolated expertise, which can be used to make more impactful decisions.
-
Portfolio optimization: AI can enhance portfolio management by dynamically adjusting allocations for new deals, similarly to quantitative funds. By analyzing historical performance, market trends, and risks, AI tools can recommend capital reallocation to optimize the balance of risk and return. This helps private equity firms maintain a diversified, data-driven portfolio and make strategic adjustments to maximize returns and manage risks.
-
Generative AI insights: Once multiple deals are in the pipeline, AI tools like GenAI can help extract tactical insights from unstructured documents such as investor communications or meeting notes. This transformation of qualitative information into quantifiable data can aid risk assessments, investor sentiment analysis, and tactical decisions for specific deals.
It’s important to remember that AI serves not as a replacement but as an interface to support human decision-making. Private equity’s evolving relationship with data focuses on improving human judgment. While trust and relationships remain vital, AI and machine learning tools can strengthen these foundations by providing more precise insights and improving investor communication.
Conclusion: the road ahead for AI in Private Equity
Looking at it from high-level, AI and data science can help private equity firms source deals more efficiently, streamline decision-making, and create more value for portfolio companies and investors across the investment lifecycle.
At Fonction Labs, we are seeing three primary categories of action for private equity: back-office operations, portfolio management, and business-wise decisions. Within business-wise decisions, deal sourcing present key areas where AI and data analytics offer promising opportunities.
In the future, AI could also further change the deal execution phase through automated due diligence. AI tools could automate the collection and verification of financial records, legal documents, and market metrics, identifying anomalies or operational inefficiencies with greater speed and accuracy than traditional methods. This would not only streamline the due diligence process but also enhance consistency and transparency.
Final thoughts
Integration of AI techniques into private equity is not about replacing human expertise but enhancing the latter. In an industry where discretion and trust are paramount, AI presents an opportunity to support and strengthen decision-making, reduce risks, and uncover previously hidden opportunities.
For private equity firms willing to embrace this shift, AI-driven deal sourcing is not just a competitive advantage—it’s a fundamental change in how firms identify and capture value.
If you are working at a PE firm and would like to discuss the topic in more details, get in touch now!