Introduction
In today’s world of abundant information, Large Language Models (LLMs) can transform how you and your teams work. Combining these AI models with data retrieval capabilities is known as RAG: Retrieval-Augmented Generation). RAG techniques can utilize information from vast corpuses and data sources as context to solve complex everyday tasks. They help optimize daily tasks and frees up precious time.
In this article, let’s discover how RAG can revolutionize your business through 3 concrete applications.
What is Retrieval-Augmented Generation (RAG)?
Retrieval-Augmented Generation is a technique based on Large Language Models (LLMs), which extends their standards scope of application by allowing them to access contextual information and documents of various types (text, image, audio, video, web links, etc.).
This allows LLMs like Chat-GPT to answer questions which they would not normally be able to. Also, RAG has the advantage of being very flexible, contrarily to other approaches like re-training or fine-tuning, which are very complex and time-consuming since they have to be performed every time the document corpus changes.
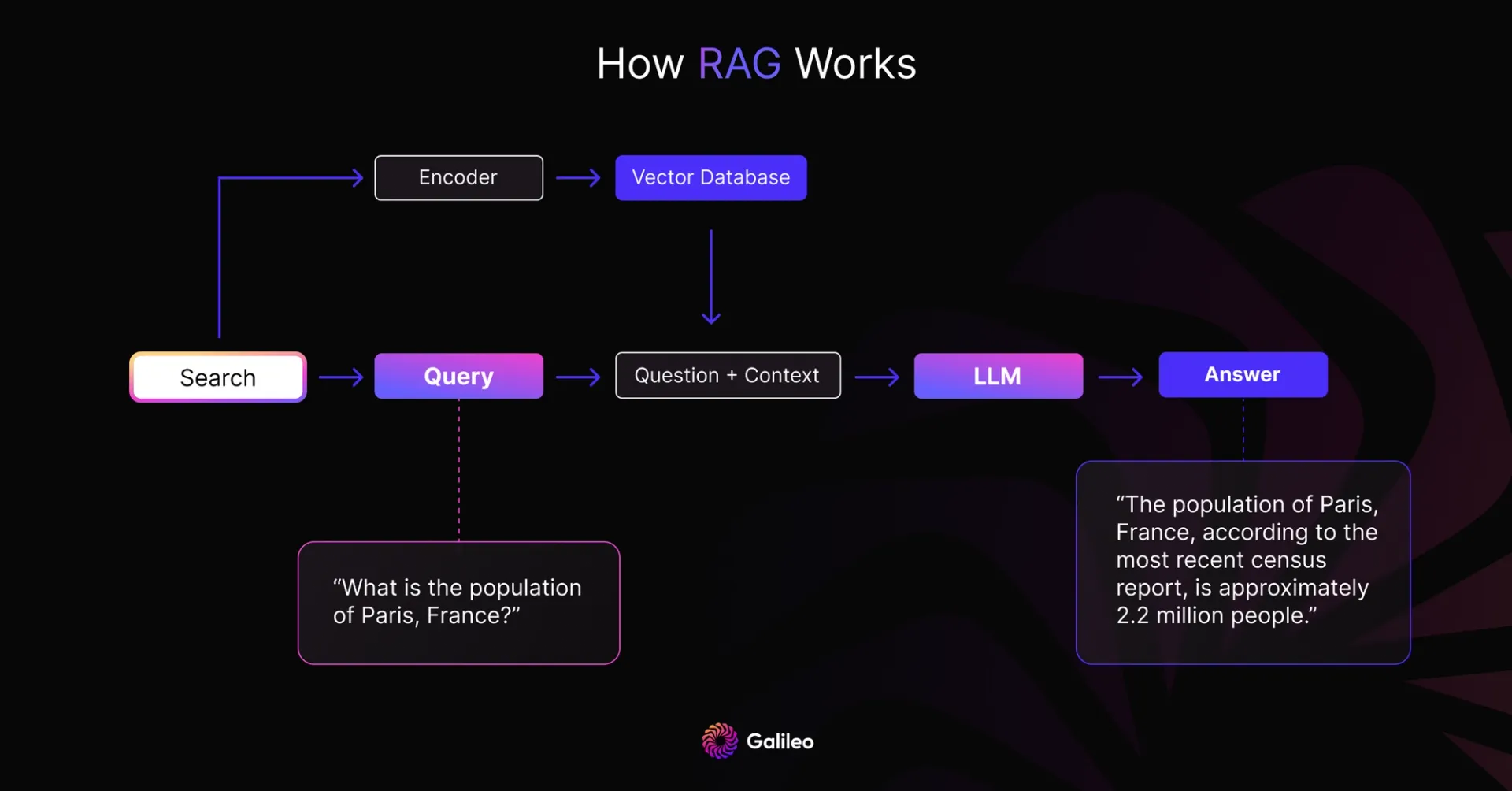
In many cases, the way this works is as follows (you may want to skip this technical explanation):
- Relevant documents are encoded in the form of vectors.
- A database of the encoded document vectors is created.
- Every time a end-user asks a question, relevant bits of the documents are retrieved using a vector-similarity between the user query and the database vectors.
- An LLM is finally prompted with the user question and retrieved context in order to get the final answer to the user question.
How RAG can help you in your every-day life as a manager
1. Optimizing information search and retrieval 🔍
Use cases: analysis of technical or legal documents, financial statements, ESG reports, etc.
If you’re a manager or executive, you probably spend hours sifting through lengthy reports or internal documentation just to find the one line with the crucial information you are seeking. An RAG model can analyze thousands of documents and find the answers to your specific questions in seconds. Imagine a virtual assistant capable of pinpointing exactly where to find the necessary data, transforming document research into a quick and efficient look-up task.
Advantages: drastic reduction in time spent on searching, allowing for a focus on analysis and strategic decision-making.
2. Automated synthesis of massive corpuses of information 📚
Use cases: automated reporting, economic intelligence, news analysis
Executives and managers need to stay constantly updated with their internal KPIs, or on external information such as the latest economic and geopolitical news. LLMs with an RAG capability can scan hundreds of articles, videos, and reports, filtering relevant information, and providing concise and accurate summaries. This way, you can always have essential information at hand without dedicating hours to it.
Advantages: efficient information synthesis and significant time savings in making informed decisions.
3. Automated report writing 📝
Use cases: writing of financial reports, administrative documents, meeting minutes (notes)
Writing reports can be a tedious and repetitive task. With LLMs, you can automate the creation of these documents. RAG models can extract the necessary information from the source data, and draft an initial version of a report that you and your employees can easily later adjust. This frees up time for higher-value tasks while ensuring consistent quality of the produced documents.
Advantages: time savings in writing, improved productivity, standardization of written reports.
Conclusion
LLM-based Retrieval-Augmented Generation (RAG) is a powerful tool for increasing your company’s efficiency. By automating information searches, content synthesis, and document writing, you can free up valuable resources and enable your teams to focus on strategic tasks.